15 best datasets for chatbot training
Also, they used three different partition protocols along with the 20 trials for better results. They used US-based National Health and Nutrition Survey data of diabetic and nondiabetic individuals and achieved promising results with the proposed technique. Ahuja et al. [20] performed a comparative analysis of various machine learning approaches, i.e., NB, DT, and MLP, on the PIMA dataset for diabetic classification. The authors suggested that the performance of MLP can be enhanced by fine-tuning and efficient feature engineering. Recently, Mohapatra et al. [21] have also used MLP to classify diabetes and achieved an accuracy of 77.5% on the PIMA dataset but failed to perform state-of-the-art comparisons. MLP has been used in the literature for various healthcare disease classifications such as cardiovascular and cancer classification [35, 36].
- It is evident from the results that our proposed calibrated MLP model could be used for the effective classification of diabetes.
- The key behind using LSTM for this problem is that the cell remembers the patterns over a long period, and three portals help regulate the information flow in and out of the system.
- So that we save the trained model, fitted tokenizer object and fitted label encoder object.
- Based on CNN articles from the DeepMind Q&A database, we have prepared a Reading Comprehension dataset of 120,000 pairs of questions and answers.
For experimental evaluation, a benchmark PIMA Indian Diabetes dataset is used. During the analysis, it is observed that MLP outperforms other classifiers with 86.08% of accuracy and LSTM improves the significant prediction with 87.26% accuracy of diabetes. Moreover, a comparative analysis of the proposed approach is also performed with existing state-of-the-art techniques, demonstrating the adaptability of the proposed approach in many public healthcare applications. In this section, we discussed the classification and prediction algorithms for diabetes prediction in healthcare. Particularly, the significance of BLE-based sensors and machine learning algorithms is highlighted for self-monitoring of diabetes mellitus in healthcare. Machine learning plays an essential part in the healthcare industry by providing ease to healthcare professionals to analyze and diagnose medical data [8–12].
Gemini’s Human Imagery Goes Astray
In this paper, a machine learning based approach has been proposed for the classification, early-stage identification, and prediction of diabetes. Furthermore, it also presents an IoT-based hypothetical diabetes monitoring system for a healthy and affected person to monitor his blood glucose (BG) level. For diabetes classification, three different classifiers have been employed, i.e., random forest (RF), multilayer perceptron (MLP), and logistic regression (LR). For predictive analysis, we have employed long short-term memory (LSTM), moving averages (MA), and linear regression (LR).
As it interacts with users and refines its knowledge, the chatbot continuously improves its conversational abilities, making it an invaluable asset for various applications. If you are looking for more datasets beyond for chatbots, check out our blog on the best training datasets for machine learning. Input to the algorithm is eight attributes enlisted in Table 3, measured from healthy and diabetic patients. The proposed LSTM-based diabetes prediction algorithm is trained with 80% of the data, and the remaining 20% is used for testing. We fine-tuned the prediction model by using a different number of LSTM units in the cell state. This fine-tuning helps to identify more prominent features in the dataset.
The stacking ensemble used four base learners, i.e., SVM, decision tree, RBF SVM, and poly SVM, and trained them with the bootstrap method through cross-validation. However, variable selection is not explicitly mentioned and state-of-the-art comparison is missing. For example, customers now want their chatbot to be more human-like and have a character. Also, sometimes some terminologies become obsolete over time or become offensive. In that case, the chatbot should be trained with new data to learn those trends.Check out this article to learn more about how to improve AI/ML models.
If you are not interested in collecting your own data, here is a list of datasets for training conversational AI. In this article, we’ll provide 7 best practices for preparing a robust dataset to train dataset for chatbot and improve an AI-powered chatbot to help businesses successfully leverage the technology. This dataset contains almost one million conversations between two people collected from the Ubuntu chat logs.
This repo contains scripts for creating datasets in a standard format –
any dataset in this format is referred to elsewhere as simply a
conversational dataset. Twitter customer support… This dataset on Kaggle includes over 3,000,000 tweets and replies from the biggest brands on Twitter. After training, it is better to save all the required files in order to use it at the inference time. So that we save the trained model, fitted tokenizer object and fitted label encoder object. The variable “training_sentences” holds all the training data (which are the sample messages in each intent category) and the “training_labels” variable holds all the target labels correspond to each training data.
You can try this dataset to train chatbots that can answer questions based on web documents. Like all machine learning models, LLMs are trained on immense datasets to recognize patterns and make predictions. Experts agree that a model’s results are only as good as the datasets it is trained on. The proposed structural design for hypothetical real-time processing and monitoring of diabetes is shown in Figure 11. The data from the user’s mobile will be transmitted in the JavaScript Object Notation (JSON) format to the Application Program Interface (API) in any language.
Treating a chatbot nicely might boost its performance — here’s why
You can download this Relational Strategies in Customer Service (RSiCS) dataset from this link. This dataset contains over one million question-answer pairs based on Bing search queries and web documents. You can also use it to train chatbots that can answer real-world questions based on a given web document. Break is a set of data for understanding issues, aimed at training models to reason about complex issues. It consists of 83,978 natural language questions, annotated with a new meaning representation, the Question Decomposition Meaning Representation (QDMR). We have drawn up the final list of the best conversational data sets to form a chatbot, broken down into question-answer data, customer support data, dialog data, and multilingual data.
This dataset contains automatically generated IRC chat logs from the Semantic Web Interest Group (SWIG). The chats are about topics related to the Semantic Web, such as RDF, OWL, SPARQL, and Linked Data. You can also use this dataset to train chatbots that can converse in technical and domain-specific language.
People attempting to get the best results out of chatbots have noticed the output quality depends on what you ask them to do, and it’s really not clear why. In August, computer scientists at the University of Toronto released CLAIRify, an interface that translates natural language instructions into a task plan for robots to execute chemistry experiments. You can foun additiona information about ai customer service and artificial intelligence and NLP. And a team from the University of California, Berkeley, trained ChatGPT to scour research papers and summarize synthesis information for making metal-organic frameworks.
Integration With Chat Applications
Goal-oriented dialogues in Maluuba… A dataset of conversations in which the conversation is focused on completing a task or making a decision, such as finding flights and hotels. Contains comprehensive information covering over 250 hotels, flights and destinations. Ubuntu Dialogue Corpus consists of almost a million conversations of two people extracted from Ubuntu chat logs used to obtain technical support on various Ubuntu-related issues. We discussed how to develop a chatbot model using deep learning from scratch and how we can use it to engage with real users. With these steps, anyone can implement their own chatbot relevant to any domain.
The data produced at this stage will be in the form of messages, which are then transferred to the Kafka application [27]. Kafka will store all the data and messages and deliver the required data and processed output to the endpoints that could be a web server, monitoring system, or a database for permanent storage. In Kafka, application data are stored in different brokers, which can cause latency issues. Therefore, within the system architecture, it is vital to consider processing the readings from the sensors closer to the place where data are acquired, e.g., on the smartphone. The latency problem could be solved by placing sensors close to the place, such as a smartphone where data are sent and received. Several attempts have also been made in the literature for diabetic prediction due to its importance in real life.
Instead, researchers could give a page of documentation or source code to a language model, which would learn how to use that tool and create a natural language interface for the researcher. “Now you can use a hundred tools, and you can still communicate your intent in natural language,” he says. It is evident from the results that our proposed calibrated MLP model could be used for the effective classification of diabetes. The proposed classification approach can also be beneficial in the future with our proposed hypothetical system.
They used random forest, logistic regression, and naïve Bayes and compared their performance with state-of-the-art individual and ensemble approaches, and their system outperforms with 79% accuracy. Malik et al. [25] performed a comparative analysis of data mining and machine learning techniques in early and onset diabetes mellitus prediction in women. They exploited traditional machine learning algorithms for proposing a diabetes prediction framework. The proposed system is evaluated on a diabetes dataset of a hospital in Germany. The empirical results show the superiority of K-nearest neighbor, random forest, and decision tree compared to other traditional algorithms. Natural Questions (NQ) is a new, large-scale corpus for training and evaluating open-domain question answering systems.
The central theme of the proposed healthcare monitoring system is the collection of data from sensors using wireless devices and transmitting to a remote server for diagnosis and treatment of diabetes. Rule-based procedures will be applied for the suggestions and treatment of diabetes, informing the patient about his current health condition, prediction, and recommendation of future changes in BG. To predict diabetes, we used moving averages with the experimental setup due to its effectiveness in diabetes prediction for children [56]. It is based on a calculation that analyzes data points by creating a series of averages of the subset of the data randomly. The moving average algorithm is based on the “forward shifting” mechanism. It excludes the first number from the series and includes the next value in the dataset, as shown in equation (3).
What is Machine Learning?
NPS Chat Corpus… This corpus consists of 10,567 messages from approximately 500,000 messages collected in various online chats in accordance with the terms of service. Yahoo Language Data… This page presents hand-picked QC datasets from Yahoo Answers from Yahoo. Then we use “LabelEncoder()” function provided by scikit-learn to convert the target labels into a model understandable form. I will define few simple intents and bunch of messages that corresponds to those intents and also map some responses according to each intent category. I will create a JSON file named “intents.json” including these data as follows. Check out this article to learn more about different data collection methods.
Further fostering transparency and collaboration, the model’s supporting code will continue to reside on the BigCode project’s GitHub page. StarCoder2 was built using responsibly sourced data under license from the digital commons of Software Heritage, hosted by Inria. StarCoder2 models share a state-of-the-art architecture and carefully curated data sources from BigCode that prioritize transparency and open governance to enable responsible innovation at scale. Depending on the dataset, there may be some extra features also included in
each example. For instance, in Reddit the author of the context and response are
identified using additional features. Note that these are the dataset sizes after filtering and other processing.
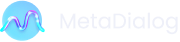
However, for the last many years, there has been a considerable emergence of chronic and genetic diseases affecting public health. Diabetes mellitus is one of the extremely life-threatening diseases because it contributes to other lethal diseases, i.e., heart, kidney, and nerve damage [3]. BigCode represents an open scientific collaboration led by Hugging Face and ServiceNow, dedicated to the responsible development of LLMs for code. Botwiki and Botmakers landing pages are all proudly hosted by , a generous supporter and the sponsor of the very first Monthly Bot Challenge.
The dataset consists of 32k task instances based on real-world rules and crowd-generated questions and scenarios. Large language models (LLMs), such as OpenAI’s GPT series, Google’s Bard, and Baidu’s Wenxin Yiyan, are driving profound technological changes. Recently, with the emergence of open-source large model frameworks like LlaMa and ChatGLM, training an LLM is no longer the exclusive domain of resource-rich companies.
arXivLabs: experimental projects with community collaborators
An absolute deficiency of insulin secretion causes type 1 diabetes (T1D). Diabetes drastically spreads due to the patient’s inability to use the produced insulin. Both types are increasing rapidly, but the ratio of increase in T2D is higher than T1D. The data used to support the findings of this study are included within the article.
As further improvements you can try different tasks to enhance performance and features. Let’s define our Neural Network architecture for the proposed model and for that we use the “Sequential” model class of Keras. You can also check our data-driven list of data labeling/classification/tagging services to find the option that best suits your project needs. If you have any questions or suggestions regarding this article, please let me know in the comment section below. MLQA data by facebook research team is also available in both Huggingface and Github.
I have already developed an application using flask and integrated this trained chatbot model with that application. To make sure that the chatbot is not biased toward specific topics or intents, the dataset should be balanced and comprehensive. The data should be representative of all the topics the chatbot will be required to cover and should enable the chatbot to respond to the maximum number of user requests.
The conversations are about technical issues related to the Ubuntu operating system. In the OPUS project they try to convert and align free online data, to add linguistic annotation, and to provide the community with a publicly available parallel corpus. QASC is a question-and-answer data set that focuses on sentence composition.
Apache Kafka will be used in real time as a delivery agent for messages in a platform that allows fault-tolerant, tall throughput, and low-latency publication. Moreover, Node.js for web design will be used as a REST API to collect sensor data. This device allows the patient to store information about BG every five minutes.
Open Assistant: Explore the Possibilities of Open and Collaborative Chatbot Development – KDnuggets
Open Assistant: Explore the Possibilities of Open and Collaborative Chatbot Development.
Posted: Thu, 27 Apr 2023 07:00:00 GMT [source]
OpenBookQA, inspired by open-book exams to assess human understanding of a subject. The open book that accompanies our questions is a set of 1329 elementary level scientific facts. Approximately 6,000 questions focus on understanding these facts and applying them to new situations. Google is battling OpenAI, whose biggest investor is Microsoft, to develop the best training models for AI systems. The engineers asked the LLM to tweak these statements when attempting to solve the GSM8K, a dataset of grade-school-level math problems.
Get a quote for an end-to-end data solution to your specific requirements. Self-driving, or automated, laboratories have been long-standing dreams for scientists working in drug and materials discovery. Much of this research is conducted through a painstaking iterative process of designing, executing, and refining experiments. AI-driven robotic labs can carry out these complex tasks without human intervention, speeding up scientific discovery and freeing time for humans to pursue creative, intellectual endeavors. Diabetes is a metabolic disorder that impairs an individual’s body to process blood glucose, known as blood sugar. This disease is characterized by hyperglycemia resulting from defects in insulin secretion, insulin action, or both [3].
Second, a linear regression model is applied to the PIMA Indian dataset with the same experimental setup. We used this approach to model a relationship between a dependent variable, that is, outcome in our case, and one or more independent variables. The autonomous variable response affects a lot on the target/dependent variable, as shown in equation (4). We use a simplified hypothesis and cost function for multivariate linear regression, as there are eight different variables in our dataset [57].
In addition, we have included 16,000 examples where the answers (to the same questions) are provided by 5 different annotators, useful for evaluating the performance of the QA systems learned. With the help of the best machine learning datasets for chatbot training, your chatbot will emerge as a delightful conversationalist, captivating users with its intelligence and wit. Embrace the power of data precision and let your chatbot embark on a journey to greatness, enriching user interactions and driving success in the AI landscape. Moreover, the proposed model will help the users to find out the risk of diabetes at a very early stage and help them gaining future predictions of their BG increase levels.
Årsand et al. [43] offered the easiest method for monitoring blood glucose, physical activity, insulin injections, and nutritional information using smartphones and smartwatches. Morón et al. [44] observed the performance of the smartphone used in the medical field. Lee and Yoo [45] anticipated a structure using PDA (personal digital assistant) to manage diabetic patient’s conditions better. Hussain and Naaz [26] presented a thorough review of machine learning models presented during 2010–2019 for diabetes prediction. They compared traditional supervised machine learning models with neural network-based algorithms in terms of accuracy and efficiency.